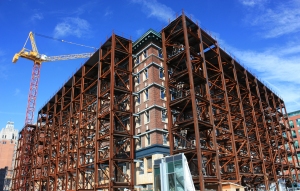
© David931 | Stock Free Images & Dreamstime Stock Photos
Monte Carlo simulations are most commonly used to understand the properties of a particular statistic such as the mean, or an estimator like maximum likelihood (ML) regression methods.
The principal is straight forward. Create a data set with a known correlation or covariance structure. Then add in some random error, and estimate your statistic or model.
Replicate this process 1,000 or 10,000 times – collecting the relevant information from each trial – and you’ll have a nice sampling distribution with which to evaluate the properties of your model or statistic.
The replication can be accomplished easily enough with a -forvalues-
loop.
In this article, you’ll find out how to accomplish the other part of the task: creating a data set with a known correlation structure.
Continue reading